
How to create 2D Gaussian noise
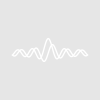
fymaterials
1/sqrt(2*pi*sigma1*sigma2)*exp[(Y1-mu1)^2/2/sigma1^2]*exp[(Y2-mu2)^2/2/sigma2^2]
Do you have any idea how to do it? I know gnoise can create 1D Gaussian noise, but it seems Igor does not have any function creating 2D Gaussian noise.
Thanks!
Arthur
I believe your Y1 and Y2 are equivalent to scaled row and columns indices which in Igor wave assignment statements are represented by x and y.
For more on wave assignment statements, execute:
and
May 12, 2011 at 10:10 pm - Permalink
In general, if you don't have a specific noise function you could use the Rejection method (see e.g., Numerical Recipes 3rd Ed. Chapter 7 or http://en.wikipedia.org/wiki/Rejection_sampling) which only requires that you have an expression for your distribution and access to enoise().
The expression that you provide corresponds to a 2D Gaussian with zero correlation between the two dimensions. As such you could obtain such distribution from a product of two distributions (one for each dimension). The extra complication is that gnoise() is centered at zero so you need to add an offset. For example, to get a Gaussian distribution centered around mu1 with standard deviation sigma1 you would use mu1+gnoise(sigma1).
It is not clear to me what you mean by a "2D variable (Y1, Y2)". I expect that the answer to that would be to create two 1D waves such that the first corresponds to Y1 and the second to Y2:
Make/O/N=(someNumber) Y1,Y2
Y1=mu1+gnoise(sigma1)
Y2=mu2+gnoise(sigma2)
In this case the normalized joint-histogram of the two waves will have the desired distribution.
A.G.
WaveMetrics, Inc.
May 13, 2011 at 10:16 am - Permalink
See see http://www.igorexchange.com/node/1373 for the JointHistogram function. If the 2D joint Gaussian variables are correlated, the procedure is more complicated, but doable.
May 13, 2011 at 11:00 am - Permalink
Note that for display purposes, I have plotted the log of the histogram values. The graph recreation procedure for displaying the histogram is (be careful with possible line wraps)
A typical graph is attached, from executing
May 14, 2011 at 06:17 am - Permalink
See Numerical Recipes or wikipedia: http://en.wikipedia.org/wiki/Cholesky_decomposition [under Monte Carlo]
John Bechhoefer
Department of Physics
Simon Fraser University
Burnaby, BC, Canada
May 20, 2011 at 07:55 pm - Permalink