
Convert linear-fit results onto an orthogonal basis
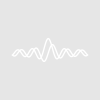
millot1
I have the results of the linear fit of some experimental data:
y=a0+a1.x
together with the covariance matrix:
sigma_square_a0,
sigma_square_a1,
sigma_a0_a1.
I d like to express the results using an orthogonal polynomial basis, that is to say as
y=b0+b1.(x-c)
with
sigma_b_c=0 and
sigma_square_c=0.
I bet it is just a matter or diagonalizing the covariance matrix but i don't see how to define the c.
Any thoughts?
In your case, with a linear fit, you just have to define your variables so that the range is symmetric about zero. Check out this code:
Then
shows that the correlation is 0 when the range is symmetric and not otherwise.
John Bechhoefer
Department of Physics
Simon Fraser University
Burnaby, BC, Canada
November 13, 2013 at 01:59 pm - Permalink
However, i do not have the original dataset.
i only have the published fit (with usual polynomial) results together with the covariance matrix.
I was wondering whether there was an analytical way of going from y=a0+a1.x (non orthogonal polynomial basis) to y=b0+b1.(x-c) (orthogonal polynomial basis)
I need to go into this particular basis because the parameters of the fit into the orthogonal polynomial basis are the input of another code.
I guess i could also "brute force" generating a "random" dataset having the covariance of the original fit results and fit it onto the new orthogonal basis. I wonder how to do the fit though, in particular how to determine c. Maybe by optimizing the chi-square ?
November 13, 2013 at 02:38 pm - Permalink