
The following functions return numbers from pseudo-random distributions of the specified shapes and parameters. Except for enoise and gnoise where you have an option to select a random number generator, the remaining noise functions use a Mersenne Twister algorithm for the initial uniform pseudo-random distribution. Note that whenever you need repeatable results you should use SetRandomSeed prior to executing any of the noise functions.
Built-in noise functions and distributions
Noise Function | Distribution |
binomialNoise | Binomial distribution |
enoise | Uniform distribution |
expNoise | Exponential distribution |
gammaNoise | Gamma distribution |
gnoise | Gaussian distribution |
HyperGNoise | Hypergeometric distribution |
logNormalNoise | Lognormal distribution |
poissonNoise | Poisson distribution |
StatsPowerNoise | Power distribution |
StatsVonMisesNoise | Von Mises distribution |
WNoise | Two-parameter Weibull distribution |
You can easily verify that the various noise functions indeed generate the desired distribution. For example, suppose you generated 10000 data points from a Gamma distribution as in:
Make/O/N=1e4 noiseWave=gammaNoise(10,2)
Make/O/N=100/O W_Hist Histogram/P/B={0,1,100} noiseWave,W_Hist
To fit this to a gamma distribution we write the following user function:
Function myFit(w,x) : FitFunc Wave w Variable x return x^(w[0]-1)*exp(-x/w[1])/((w[1]^w[0])*Gamma(w[0])) End
To determine the initial guesses for the fitting coefficients we note that the average of noiseWave is 19.8681. This average should be equal to the product a*b of the two parameters of the gamma distribution. Our guess is therefore a=b=sqrt(19.8)
Make/O/D/N=2 W_coef={4.48,4.48} FuncFit/NTHR=0/TBOX=768 myFit W_coef W_Hist[1,50] /D
The resulting fit to a Gamma distribution is drawn below in blue plotted over the calculated distribution (red).

Forum

Support
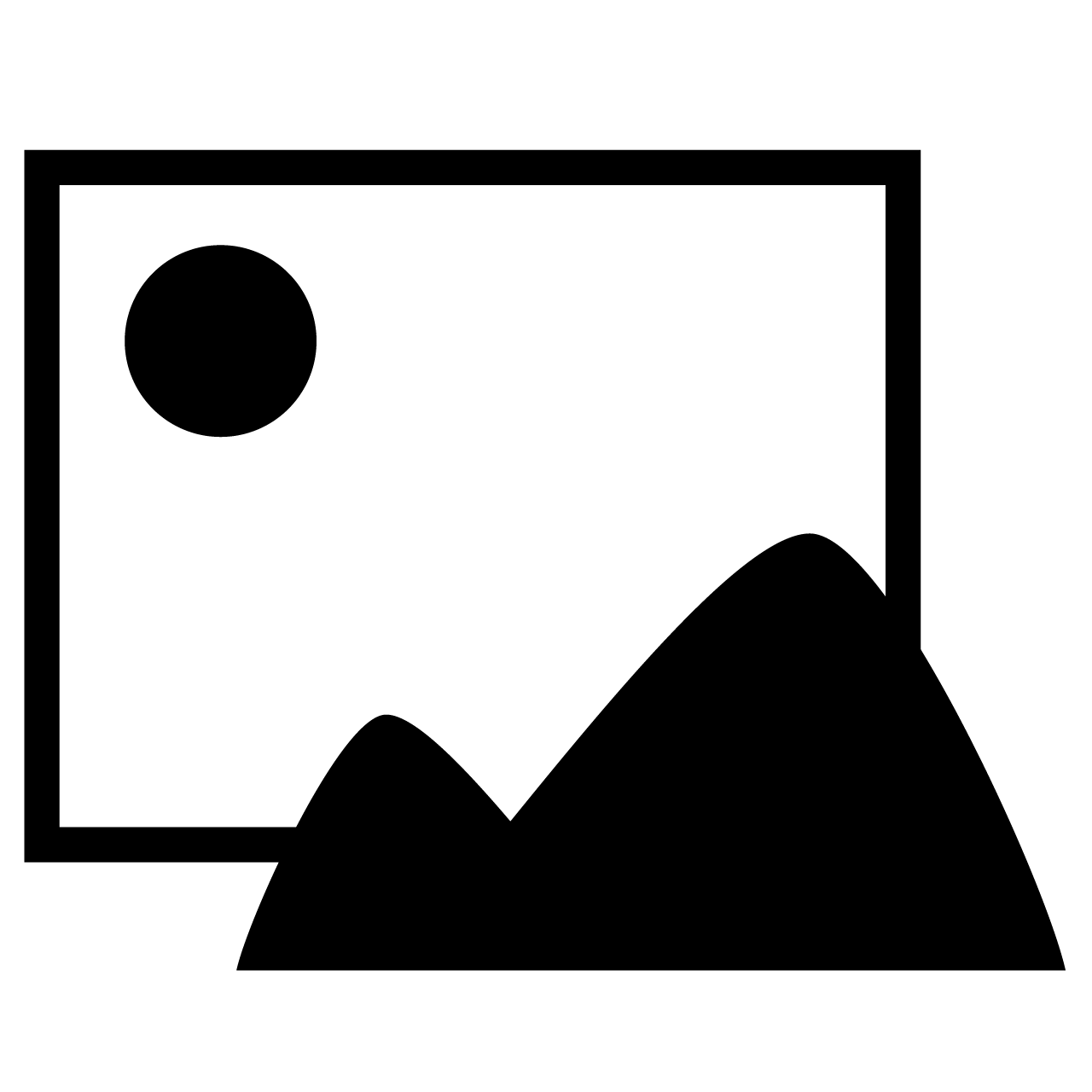
Gallery
Igor Pro 9
Learn More
Igor XOP Toolkit
Learn More
Igor NIDAQ Tools MX
Learn More